Details
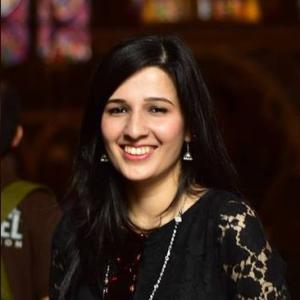
- Affiliation
-
AffiliationUniversity College Dublin
- Country
-
CountryIreland
In this article, non-uniformly sampled electrocardiogram (ECG) signals obtained from level-crossing analog-to-digital converters (LC-ADCs) are analyzed for event-driven classification and compression performance. The signal compression results show that it is important to assess the distortion in event-driven signals when simulating LC-ADC models, especially at lower resolutions and larger quantization steps. The effects of varying the LC-ADC parameters for the application of cardiac arrhythmia classifiers are also assessed using an artificial neural network (ANN) and the MIT-BIH Arrhythmia Database. In comparison with uniformly-sampled data, it is possible to achieve comparable classification accuracy at a much lower complexity with event-driven ECG signals. The results show the best event-driven model achieves over 97% accuracy with 79% reduction in ANN complexity with signal-to-distortion ratio (S/D)≥21dB. For S/D<21dB, the best event-driven model achieves 93% accuracy with a 96% reduction in ANN complexity. An open-source event-driven arrhythmia database is also presented.