Details
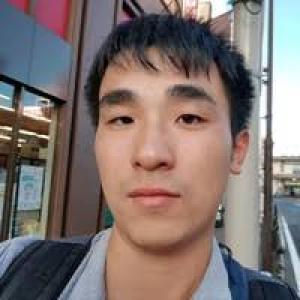
- Affiliation
-
AffiliationThe University of Edinburgh
- Country
-
CountryUnited Kingdom
Edge AI accelerators are being targeted for numerous emerging applications due to their compact size and low power consumption. However, these applications require reliability, fault-tolerance, and flexibility to overcome the defect rate caused by radiation or manufacturing defects for hard-to-reach applied environments such as space or nuclear power stations. As a result, this paper proposes a dynamically reconfigurable structure for the column streaming-based convolution engine in order to enhance the edge AI accelerator’s reliability and flexibility. The high-level description code synthesized from Xilinx Vivado software shows that the design offers more mapping methods in terms of flexibility with 17.15% powerless and [0.43%, 3.18%] area overhead as a trade-off compared to the commercial streaming-based CNN accelerator.