Details
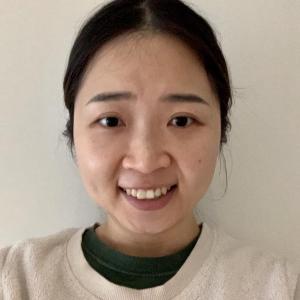
- Affiliation
-
AffiliationUniversity College London
- Country
A real-time discrete wavelet transform-based adaptive voice activity detector and sub-band selection for feature extraction are proposed for noise classification, which can be used in a speech processing pipeline. The voice activity detection and sub-band selection rely on wavelet energy features and the feature extraction process involves the extraction of mel-frequency cepstral coefficients from selected wavelet sub-bands and mean absolute values of all sub-bands. The method combined with a feedforward neural network with two hidden layers could be added to speech enhancement systems and deployed in hearing devices such as cochlear implants. In comparison to the conventional short-time Fourier transform-based technique, it has higher F1 scores and classification accuracies (with a mean of 0.916 and 90.1%, respectively) across five different noise types (babble, factory, pink, Volvo (car) and white noise), a significantly smaller feature set with 21 features, reduced memory requirement, faster training convergence and about half the computational cost.