Details
Poster
Presenter(s)
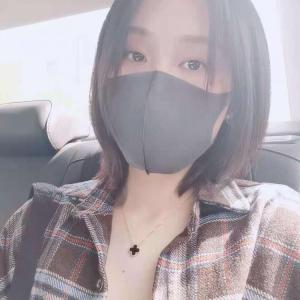
Display Name
Yuqi Zhu
- Affiliation
-
AffiliationAcademy of Military Science
- Country
-
CountryChina
Abstract
Pommerman is a recently-proposed multi-agent benchmark. The main obstacles are the delayed action effects and sparse reward. This paper presents approaches to mitigate these problems by introducing Artificial Potential Field in Pommerman. We propose a new framework to generate hybrid features from both APF computation and raw environment data. Meanwhile, a new reward shaping method through APF is developed to give the agent a faster and more efficient policy iteration. The training results show that the learning speed and convergence reward are both improved on a 1v1 mode of Pommerman game, compared to the conventional learning algorithms, A2C and ACKTR.