Details
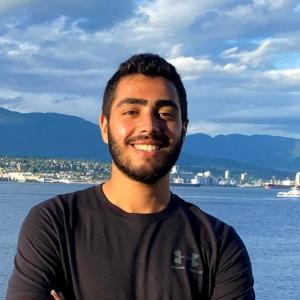
- Affiliation
-
AffiliationPolytechnique Montréal
- Country
This paper proposes a methodology based on machine learning to find apparent causal relations between performance targets and design variables in analog circuits. Diversified filtering and wrapping variable selection algorithms are utilized to construct a causal graph that identifies the major circuit design parameters that can be used to optimize the performance of analog circuits. Based on the constructed causal graph, a sequence of design procedures can be extracted and followed to optimize the performance of a design. The proposed methodology is validated using a two-stage op-amp. The obtained causal graph agrees with analytical design equations published in the literature for the selected two-stage op-amp. The results also show that the proposed methodology can accelerate the circuit design process and effectively help designers understand the reasoning behind different design decisions.