Details
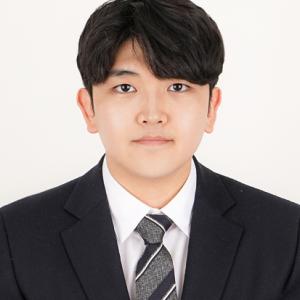
- Affiliation
-
AffiliationDaegu Gyeongbuk Institute of Science and Technology
- Country
In this paper, we present a novel approach in developing input-to-neuron interlinks to achieve better accuracy in spike-based liquid state machines. An energy-efficient spike-based neural networks suffer from lower accuracy in image classification compared to deep learning models. The previous LSM models randomly connect input neurons to excitatory neurons in a liquid. This limits the expressive power of a liquid model as large portion of excitatory neurons become inactive which never fire. To overcome this limitation, we propose an adaptive interlink development method which achieves 3.2% higher classification accuracy than the static LSM model. Also, our hardware implementation on FPGA improves performance by 3.16∼4.99x or 1.47∼3.95x over CPU/GPU.