Details
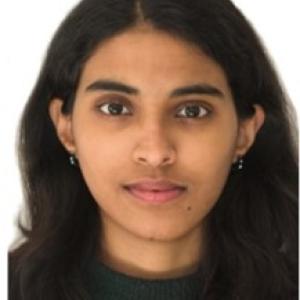
- Affiliation
-
AffiliationUniversity College Dublin
- Country
Internet of Things (IoT) enabled wearable sensors for health monitoring are widely used to reduce the cost of personal healthcare and improve quality of life. The sleep apnea-hypopnea syndrome greatly affects the quality of sleep of an individual. This work introduces a novel method for apnea detection from electrocardiogram (ECG) signals obtained from wearable devices. The novelty stems from the high resolution of apnea detection on a second-by-second basis, and this is achieved using a 1-dimensional convolutional neural network for feature extraction and detection of sleep apnea events. The proposed method exhibits an accuracy of 99.56% and a sensitivity of 96.05%. The pruned model with 80% sparsity exhibited an accuracy of 97.34% and a sensitivity of 86.48%. The binarized model exhibited an accuracy of 75.59% and sensitivity of 63.23%. The patient-specific models on average exhibited an accuracy of 97.79% and sensitivity of 92.23%.