Details
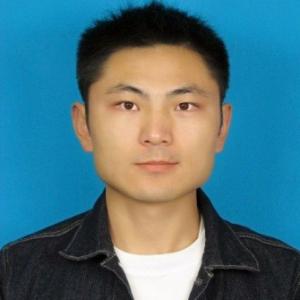
- Affiliation
-
AffiliationShanghai Jiao Tong University
- Country
With increased number of simultaneous neural recording channels, data compression with high fidelity and low hardware cost is necessary to reduce bandwidth requirement and overall power consumption. Fully event-based neural spike sorting has activity dependent workload without constant clock signal and shows high power efficiency. This paper describes an unsupervised Spike Sorting system, aiming a fully clockless operation without external intervention. With improved spike detection and feature extraction in continuous time (CT) domain. Unsupervised detection and sorting method are proposed and implemented in the CT domain, and sorting accuracy shows improvement along the self-calibration process. The design was verified by unsupervised spike Sorting among data sets with different signal-to-noise ratio (SNR), the sorting accuracy can exceed 90\\% after 16 spikes at a SNR of 0dB.