Details
Poster
Presenter(s)
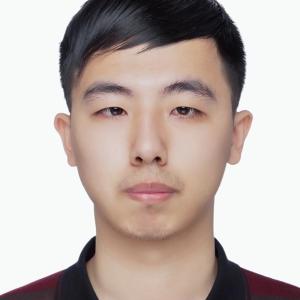
Display Name
Xiaoyu Feng
- Affiliation
-
AffiliationTsinghua University
- Country
Abstract
Compression techniques like pruning and quantization have been proven effective for accelerators' energy efficiency. However, compression has always been difficult and time-consuming. This paper proposes a reinforcement learning (RL) based joint compression framework to find the appropriate pruning ratio and quantization bandwidth for accelerators. By interacting with an energy model of the target accelerator, the RL agent can find an optimal compression scheme after the trial-and-error process. Compared with separate pruning and quantization, the proposed framework can achieve at least $25%$ energy reduction or higher accuracy. The framework can achieve $90%$ and $85%$ energy reduction on Cifar10 and Cifar100.