Details
Poster
Presenter(s)
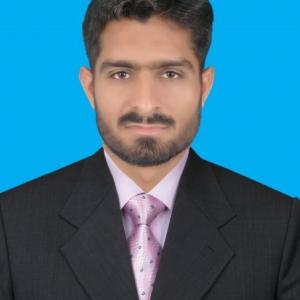
Display Name
Muhammad Ijaz
- Affiliation
-
AffiliationHamad Bin Khalifa University
- Country
Abstract
The drift of gas sensors is a challenging issue that limits the use of sensors over longer periods of time because the pattern recognition and classification systems fail to recognize the deviated response of sensors. To address this problem, this paper proposes the use of Recursive Feature Elimination (RFE) based Random Forests (RF) for compensation of small-scale drift in gas sensors. The proposed method is evaluated for the classification of six volatile compounds and is compared with multiple state-of-the-art classifiers and feature selection techniques using a benchmark dataset publicly available online. The results depict that the RF-RFE combination outperforms the other classifiers and feature selection techniques.