Details
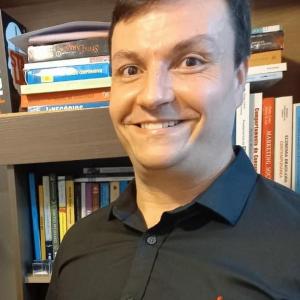
- Affiliation
-
AffiliationFederal University of Pelotas (UFPel)
- Country
Deep learning-based side-channel attacks (DL-SCA) has drawn significant interest in academic research. Despite the significantly superior results in practice, very few effort have been made in order to reduce time-consuming process of DL-SCA. The difficulty to define optimal hyperparameters and to train deep neural networks may become a serious limitation for their practical applications. Therefore, in this paper, we propose an improved pruning-based surgery as a way to reduce the size of neural networks, decreasing training time while keeping the profiling attack performance, especially on targets with countermeasures. The results show that the proposed method is 9 times faster for the MLP network and 6.38 times for the CNN network compared to the original surgery method applied to SCA.