Details
Poster
Presenter(s)
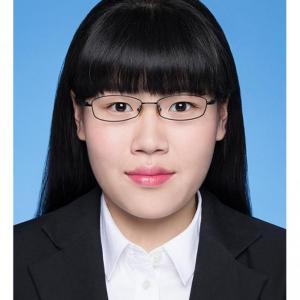
Display Name
Weijing Wen
- Affiliation
-
AffiliationFudan University
- Country
Abstract
In this work, we propose a novel regularization method to learn hardware-friendly sparse structures for deep recurrent neural networks, where low-rank structured sparse approximations of the weight matrices are learned through the regularization without dimension distortion. Experiments on language modeling of Penn TreeBank, enwik8 and text8 datasets show that our approach can achieve comparable perplexity with higher sparsity than the state-of-the-art structured sparsity learning method.