Details
Poster
Presenter(s)
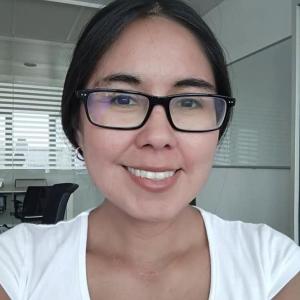
Display Name
Lizeth Gonzalez-Carabarin
- Affiliation
-
AffiliationEindhoven University of Technology
- Country
-
CountryNetherlands
Abstract
Wearable solutions based on Deep Learning (DL) for real-time ECG monitoring are a promising alternative to detect life-threatening arrhythmias. However, DL models suffer of a large memory footprint, which hampers their adoption in portable technologies. Therefore, we leverage a hardware-oriented pruning approach to effectively shrink DL models. We demonstrate that tiny DL models can be reduced to 5.55x (pruning), and 26.6x (pruning+quantization) compression rate, with 82.9\\% FLOP\'s reduction. These ultra-compressed models are able to effectively classify life-threatening arrhythmias with minimal or no loss of performance compared with their non-pruned counterparts, which can pave the path towards DL-based biomedical portable solutions.