Details
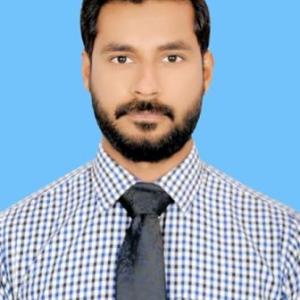
- Affiliation
-
AffiliationUniversity of Glasgow, UK
- Country
With the ever-increasing internet of things (IoT) and the rise of edge computing, federated learning (FL) is considered a promising solution for privacy and latency-aware applications. However, the data is highly distributed among several clients, making it challenging to monitor node anomalies caused by malfunctioning devices or any other unforeseen reasons. In this paper, we propose FedClamp, an anomaly detection algorithm based on the hidden Markov model (HMM) in the FL environment. FedClamp identifies the anomalous node and isolates them before aggregation to improve the performance of the global model. FedClamp was tested in a short-term energy forecasting problem using artificial neural networks when the FL environment had fie clients. The algorithm uses mean absolute percentage error (MAPE) generated from local models and clusters them in normal and faulted nodes using HMM. The anomalous nodes identified using this algorithm are isolated before aggregation and achieve global model convergence with few communication rounds.