Details
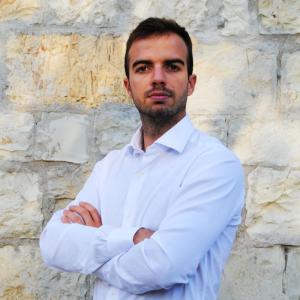
- Affiliation
-
AffiliationPolitecnico di Milano
- Country
-
CountryItaly
Drug repurposing is more relevant than ever due to drug development\'s rising costs and the need to respond to emerging diseases quickly. Knowledge graph embedding enables drug repurposing using heterogeneous data sources combined with state-of-the-art machine learning models to predict new drug-disease links in the knowledge graph. As in many machine learning applications, significant work is still required to understand the predictive models\' behavior. We propose a structured methodology to understand better machine learning models\' results for drug repurposing, suggesting key elements of the knowledge graph to improve predictions while saving computational resources. We reduce the training set of 11.05% and the embedding space by 31.87%, with only a 2% accuracy reduction, and increase accuracy by 60% on the open ogbl-biokg graph adding only 1.53% new triples.