Details
Poster
Presenter(s)
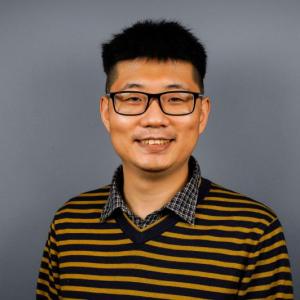
Display Name
Jinwei Zhao
- Affiliation
-
AffiliationQV Bioelectronics
- Country
Abstract
Due to their high efficiency, photovoltaic (PV) cells can power the Internet of Things (IoT) devices, including sensors, actuators, and communication devices. Generally, PV cells are connected in series to obtain a greater voltage without losing energy and active area. However, a series connection is unstable, and any fault in an array inevitably leads to a breakdown of the branch or even the system. Therefore, fault detection is essential. This study presents a systematic review of stat-of-the-art fault diagnosis methods (FDMs) of PV cells. We categorise, evaluate and summarise the fault detection methods into three broad areas: physical, threshold and artificial intelligence (AI) techniques.