Details
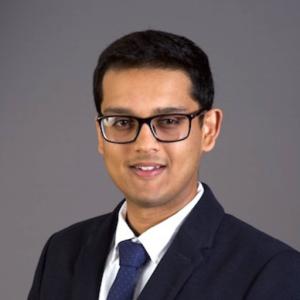
- Affiliation
-
AffiliationUniversity of California, San Diego
- Country
This work presents precise attention state classification techniques based on data recorded from a fabricated in-ear EEG instrument used during a vigilance task experiment. We recorded both on-scalp and in-ear EEG signals from multiple subjects, and we show that in-ear EEG offers comparable classification accuracy. We demonstrate 90--95\\% accuracy in classifying attentive and resting states without any need for sophisticated pre-processing or feature extraction. We also show our approach to be hardware-centric for low-power on-chip classification and contain the capability of few-shot learning, which is extremely necessary for resource-constrained applications and continuous adaptation to different subjects and various operating environments. This study suggests the future viability of a user-generic and portable integrated System-on-Chip (SoC) device for closed-loop cognitive state monitoring and neuro-feedback to enhance safety and efficiency during important daily-life tasks.