Details
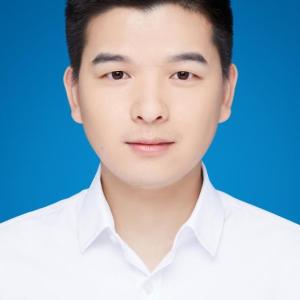
- Affiliation
-
AffiliationHangzhou Dianzi University
- Country
Hierarchical feedforward neural network trained by extreme learning machine (HELM) has been recently developed for complex and high-dimensional data learning. Although the HELM shows better generalization performance with lower computational complexity than several deep neural networks (DNNs), it is found that when the network becomes deeper, the data distribution of each layer may move to the saturated regime of the non-linear activation function, which may lead to poor generalization performance. In this paper, an affine transformation (AT) activation function based on the maximum entropy principle (MEP) is developed to achieve uniform data distribution after mapping in the autoencoder (AE) and the hidden node of last classification layer. The resultant hierarchical neural network is called the AT based HELM (AT-HELM). Experiments on 29 benchmark datasets are carried out to demonstrate the superiority of AT-HELM.