Details
- Affiliation
- Country
-
CountryChina
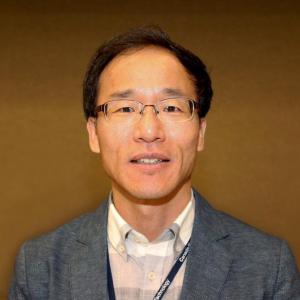
- Affiliation
-
AffiliationUniversity of Saskatchewan
- Country
Posit is designed as an alternative to IEEE 754 floating-point format for many applications. With the same bit-width, it can provide a much larger dynamic range than IEEE floating-point format. Therefore, for applications where the dynamic range of numbers is important, posit can provide an efficient solution. In addition, posit has non-uniformed number distribution. This distribution fits well with the data distribution of deep learning and signal processing applications. Therefore, posit format can provide a more efficient way to represent data in such applications. Due to these advantages, posit format is nowadays popular in many applications and one of them is deep learning. In recent years, more and more posit based deep learning hardware accelerators appear in the literature. However, due to the dynamic component bit-width of posit format, the costs of current posit arithmetic units are more expensive than floating-point units. Potentially, there are many optimizations that can be investigated specifically for posit arithmetic to reduce their hardware cost. In addition, although posit is popular for deep learning, there are still many works to do to make the posit environment complete. One example is that pure posit training of deep learning models is still absent from the literature. In this overview lecture, the basics of posit arithmetic and the current status of its applications in deep learning computation will be discussed. Then the trends and challenges of posit based deep learning will be discussed to motivate more related research works. We believe this will be a hot and timely topic within the interest of ISCAS participants. On one hand, designing power and energy efficient deep learning hardware processors is a hot topic in IEEE-CAS. The use of posit will help in the exploration of more power efficient solution for deep learning processing. On the other hand, the optimization of posit arithmetic is of interest for many ISCAS participants and the optimization also needs the contributions from ISCAS participants.
Chairs
- Affiliation
-
AffiliationKyung Hee University
- Country